A monsoon is a seasonal reversal in the prevailing wind direction, that is usually initiated by the land sea temperature contrast. The Indian summer monsoon, for example, is triggered when the land gets heated up more than the surrounding sea during the summer creating a pressure gradient between the land and the sea (Figure 1). It is well known that the ocean thermal energy required for fueling monsoon circulations comes from the upper layer of the ocean (e.g. Venugopal et al. 2018). But such amount of energy does not come from the top thin layer represented by sea surface temperature (SST) alone. Nevertheless, often SST does not represent the thermal energy available in the upper ocean, although this parameter has been the only oceanographic input to the cyclone and monsoon atmospheric numerical and statistical models.
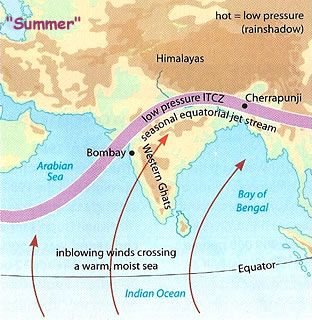
Oceanography textbooks, such as the one by Pickard and Emery (1990), point out that rapid (of the order of a day to a month) heating (such as strong solar heating) or cooling events (such as more evaporation due to strong winds and/or clouds) can quickly erase the thermal signature of subsurface warm or cold features, leading to SST misrepresenting the ocean thermal energy. This is even evident from the average coefficient of variation, defined as the relative magnitude of the standard deviation to the average value, (Figure 2) for monthly SST (0.02) 1993–2017 being double that for OMT (0.01) for the North Indian Ocean.
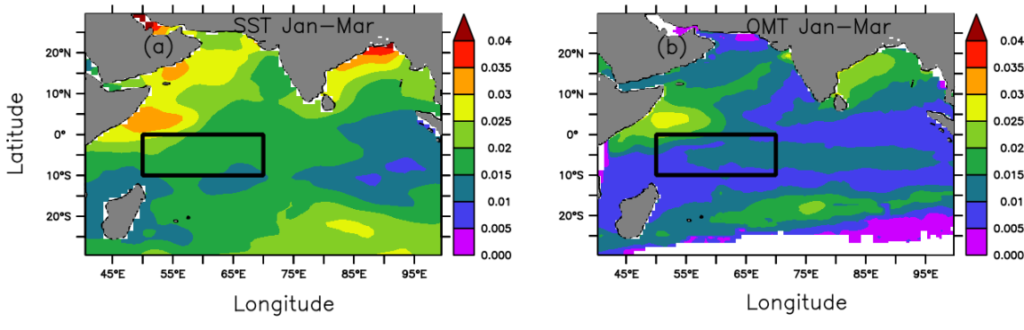
Besides, the rate of intensification and final intensity of cyclones are sensitive to the initial spatial distribution of the mixed layer, a proxy for ocean thermal energy, rather than to SST alone (Mao et al. 2000). Shay et al. (2000), Ali et al. (2007), Mainelli et al. (2008), Ali et al. (2013) and Lin et al. (2013) and Jaimes and Shay, (2015) demonstrated/suggested the importance of ocean thermal energy in cyclone studies.
Similarly, Smith et al. (1995), Ji et al. (1997) and Latif et al. (1998) proved that even the El Niño forecast models could be improved by initializing the models with the observed ocean heat content (OHC). OHC is the amount of thermal energy available in the oceans from surface to a fixed depth, say, 100m or 200m given by equation (1). Although in situ temperature profiles are required to estimate this parameter, it can be indirectly inferred from the satellite-derived sea surface height anomaly (SSHA) and SST.

where ρ is the density of the sea water, Cp the specific heat capacity of the sea water at constant pressure, p; h1 the top depth, h2 the bottom depth, dz the thickness of the layer and T is the average temperature of the layer in oC.
However, investigations using this energy for monsoon prediction have been limited. For example, Rajeevan and McPhaden (2004) reported that the relationship between warm water volume, which can be considered as a proxy to OHC, in the tropical Pacific and El Niño Southern Oscillation has a much stronger lead-time than the El Niño Southern Oscillation-SST relationship in predicting Indian summer monsoon rainfall (ISMR). Nagamani et al. (2016) reported that the OHC from the surface down to the depth of 26oC isotherm (D26) also known as Tropical Cyclone Heat Potential (TCHP), estimated using in situ, model and satellite observations, in the Arabian Sea Mini Warm Pool region (the region in the southeastern Arabian Sea from 4° to 14°N and 68° to 78°E) increased during 1998-2010 (Figure 3). They interpreted this increase as responsible for the overall decreasing trend in ISMR during this period. Lopez et al. (2016) related the variability of the heat content/transport in the South Atlantic and variability of monsoon at global scales.
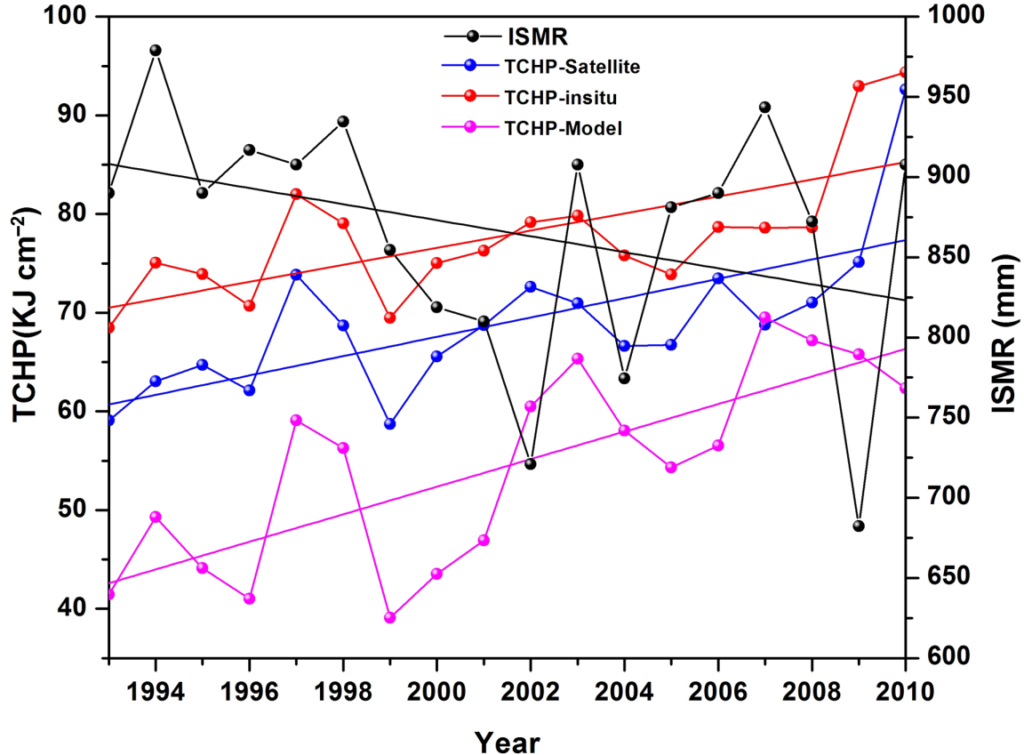
The above studies highlight the application of OHC for El Niño, tropical cyclones and monsoon studies. Since OHC/TCHP has dimensional inequality with SST, it cannot be used in place of SST in numerical models. Already Price (2009) using an ocean model, argued that a depth-averaged temperature (H100) is a more robust metric of hurricane-ocean interaction than even is OHC.
Hence, Ali et al. (2015) converted TCHP to Ocean Mean Temperature (OMT) of the surface to D26 ocean layer. They used the satellite-derived TCHP and D26, from the National Oceanic and Atmospheric Administration, Atlantic Oceanographic and Meteorological Laboratory, to estimate OMT (equation 3). TCHP is given by (Leipper and Volgenau, 1972):

Assuming the average temperature of the ocean from surface to D26 as OMT, they converted equation (2) as

Since OMT is derived from TCHP, the correlation between ISMR and OMT should be same as that between ISMR and TCHP. However, by converting TCHP to OMT, SST can be easily replaced with OMT, both having the same dimensions. Ali et al. (2015) studied the relationships of ISMR with OMT and SST at each 2.5° box in the North Indian Ocean for different months and concluded that OMT in the southwestern Indian Ocean region (50°E–70°E and 10°S–0°N; delimited by the rectangular boxes in Fig. 2) has the highest correlation with ISMR. They also reported that the correlations and confidence levels are greater for OMT compared to SST. However, they estimated only one correlation coefficient for each 2.5° box for the entire study period of 1993–2013 but did not study the performance of OMT for year-to-year ISMR variations.
Hence, Venugopal et al. (2018) focused on the skill of predicting the ISMR anomaly for different years by considering the same southwestern Indian Ocean region as in Ali et al. (2015) to explore the improvements in the predictability of ISMR year to year variations using OMT of this region instead of SST, in a statistical sense. Since the scales of ISMR and OMT/SST are different, they converted all these parameters as percentage deviations (PD) and concluded that the Jnauary-March PDs of OMT correlate better with ISMR compared to those of SST (Figure. 4).
The success rate of OMT PD averaged over January to March, is 80% while that of SST PD is 60%. For 2018 and 2019, as well, OMT predicted ISMR correctly and for 2020 the ISMR prediction using OMT is above average rainfall of 887.5 mm (thanks to Venugopal for providing predictions during 2018-2020). Predicting the sign of anomalies alone is also important because it is linked to more/less rainfall, which is critical for the agricultural economy of a country, such as India and this method predicts only above or below average rainfall without going into further classification or estimating the percentages. Other January–March mean climate indices such as NINO3.4, Indian Ocean Dipole Mode Index and El Niño Southern Oscillation Modoki Index have less predictability of 52%, 48%, and 56%, respectively. Thus, OMT has emerged out as a potential parameter for a better monsoon forecasting. However, it should be clarified that OMT would be slightly cooler than SST by about 0.5 – 1.0 0C. This difference does not make an impact in a statistical sense but certainly affects the dynamical or numerical models. However, when a relationship is statistically established, it should be possible to prove the same relationship in numerical models as well with a slight adjustment for the bias between SST and OMT. Many dynamical numerical models have problems in correctly simulating monsoons because they do not correctly simulate sea surface cooling because of several factors such as, but not limited to, (i) they do not simulate the mixed layer dynamics, (ii) are not initialized by correct SST fields and/or (iii) do not include realistic initial ocean mesoscale eddy features. By using OMT instead of SST some of these problems could be resolved at least to some extent. Secondly, since OMT is derived from sea surface height anomaly that reflects the oceanic mesoscale eddies as well, the effect of eddies is indirectly taken care of by OMT.
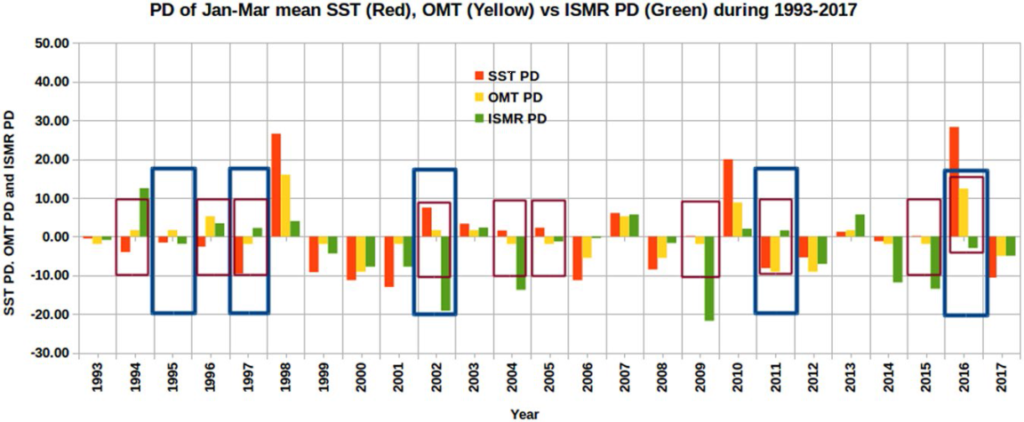
However, any alternative concept would be difficult to be accepted by the community easily, which is primarily a mindset problem. For example, at the beginning of the remote sensing applications era, satellite oceanography was not accepted. Ewing (1964) said: “Oceanography from a satellite – the words themselves sound incongruous and to a generation of scientists accustomed to Nansen bottles and reversing thermometers, the idea may seem absurd. This is all the more so because decades of technological constraint have all but forced oceanographers into consideration of the class of problems that derive from the vertical distribution of properties at stations widely separated in space and time”.
In this context, a concise, parodical statement could be: “For a meteorologist using SST as the only oceanographic input, use of OMT for atmospheric studies sounds incongruous!” This is because all the flux computations use SST as the input and many atmospheric scientists, even today, feel that the atmosphere interacts only through SST representing a thin surface layer, the required energy comes from the upper surface layer, represented by OMT though. So much heat is available in the upper layer of the ocean that, as the surface temperature cools due to the net heat loss, heat from below wells up feeding the atmosphere with the required energy.
I am sure that if our inhibitions are put aside and start using OMT instead of SST, particularly in atmospheric models, we should be able to predict the ISMR more accurately. However, I do not claim that all the intricacies of predicting the monsoon can be resolved by OMT alone!
References:
Ali, M. M., P. V. Nagamani, N. Sharma, R. T. Venu Gopal, M. Rajeevan, G. J. Goni and M. A. Bourassa, Relationship between ocean mean temperatures and Indian summer monsoon rainfall. Atmospheric Science Letters 16(3), 408–413 (2015).
Ali, M. M., P. S. V. Jagadeesh and S. Jain, Effects of eddies on Bay of Bengal cyclone intensity. Eos, Transactions American Geophysical Union 88(8): 93–95 (2007).
Ali, M. M., T. Kashyap and P. V. Nagamani, Use of sea surface temperature for cyclone intensity prediction needs a Relook. Eos, Transactions American Geophysical Union 94, 177–178 (2013).
Ewing, C. C. Oceanography from Space: Proceedings of Conference on the Feasability of Conducting Oœanographic Explorations from Aircraft, Manned Orbital and Lunar Laboratories : Held at Woods Hole, Massachusetts, 24- 28 August (1964).
Jaimes, B. and L.K. Shay, Enhanced wind-driven downwelling flow in warm oceanic eddy features during the intensification of tropical cyclone Isaac (2012): Observations and theory. J. Phys. Oceanogr. 45, 1667–1689. https://doi.org/10.1175/JPO-D-14-0176.1 (2015).
Ji, M. and A. Leetmaa, Impact of data assimilation on ocean initialization and El Nino prediction. Monthly Weather Review 125(5), 742–753 (1997).
Latif, M., D. Anderson, T. Barnett, M. Cane, R. Kleeman and A. Leetmaa, E. Schneider, A review of the predictability and prediction of ENSO. Journal of Geophysical Research: Oceans 103(C7), 14375–14393 (1998).
Leipper, D. and D. Volgenau, Hurricane heat potential of the Gulf of Mexico,
Journal of Physical Oceanography, 2, 218-224 (1972).
Lin, I. I., G. J. Goni, J. A. Knaff, C. Forbes and M. M. Ali, Ocean heat content for tropical cyclone intensity forecasting and its impact on storm surge. Natural Hazards 66(3), 1481–1500 (2013).
Lopez, H., S. Dong, S.-K. Lee and G. J. Goni, Decadal modulations of interhemispheric global atmospheric circulations and monsoons by the south Atlantic meridional overturning circulation. J. Clim. 29(5),1831-1851 (2016).
Mainelli, M., M. DeMaria, L. K. Shay and G. J. Goni, Application of oceanic heat content estimation to operational forecasting of recent Atlantic category 5 hurricanes. Weather and Forecasting 23(1), 3–16 (2008).
Mao, Q., S. W. Chang and R. L. Pfeffer, Influence of large- scale initial oceanic mixed layer depth on tropical cyclones, Mon. Weather Rev. 128, 4058–4070 (2000).
Nagamani, T. M., MM Ali, G. J. Goni, T. V. S. Udaya Bhaskar, J. McCreary, R. Weller, V. V. Gopalakrishna and J. C. Puzzello, Heat content of the Arabian Sea mini warm pool is increasing. Atmospheric Science Letters 17(1), 39-42 (2016).
Pickard, G. L. and W. J. Emery, Descriptive physical oceanography: An introduction. Elsevier (1990).
Price, J. F., Metrics of hurricane-ocean interaction: Vertically-integrated or vertically-averaged ocean temperature?, Ocean Science 5, 351–368 (2009).
Rajeevan, M. and M. J. McPhaden, Tropical Pacific upper ocean heat content variations and Indian summer monsoon rainfall. Geophysical Research Letters 31(18) (2004).
Shay, L. K., G. J. Goni and P. G. Black, Effects of a warm oceanic feature on Hurricane Opal, Mon.Weather Rev. 128, 1366–1383 (2000).
Smith, T. M., A. G. Barnston, M. Ji and M. Chelliah, The impact of Pacific Ocean subsurface data on operational prediction of tropical Pacific SST at the NCEP. Weather and forecasting 10(4), 708–714 (1995).
Venugopal, T., M.M. Ali, M. A. Bourassa, Y. Zheng, G. J. Goni, G.R. Foltz and M. Rajeevan, Statistical evidence for the role of southwestern Indian Ocean heat content in the Indian summer monsoon rainfall, Scientific Reports,r 8:12092, DOI:10.1038/s41598-018-30552-0 (2018).
Dr. MM Ali is a Ph.D. from Andhra University, India. He worked in Indian Space Research Organisation as a Scientist and is a Senior Scientist (Courtesy) at Center for Ocean-Atmospheric Prediction Studies (COAPS), Florida State University, USA. Presently he is a consultant meteorologist at Andhra Pradesh State Disaster Management Authority, India. His present research interest is in the investigation of ocean thermal energy derived from remote sensing observations for cyclone and monsoon predictions.